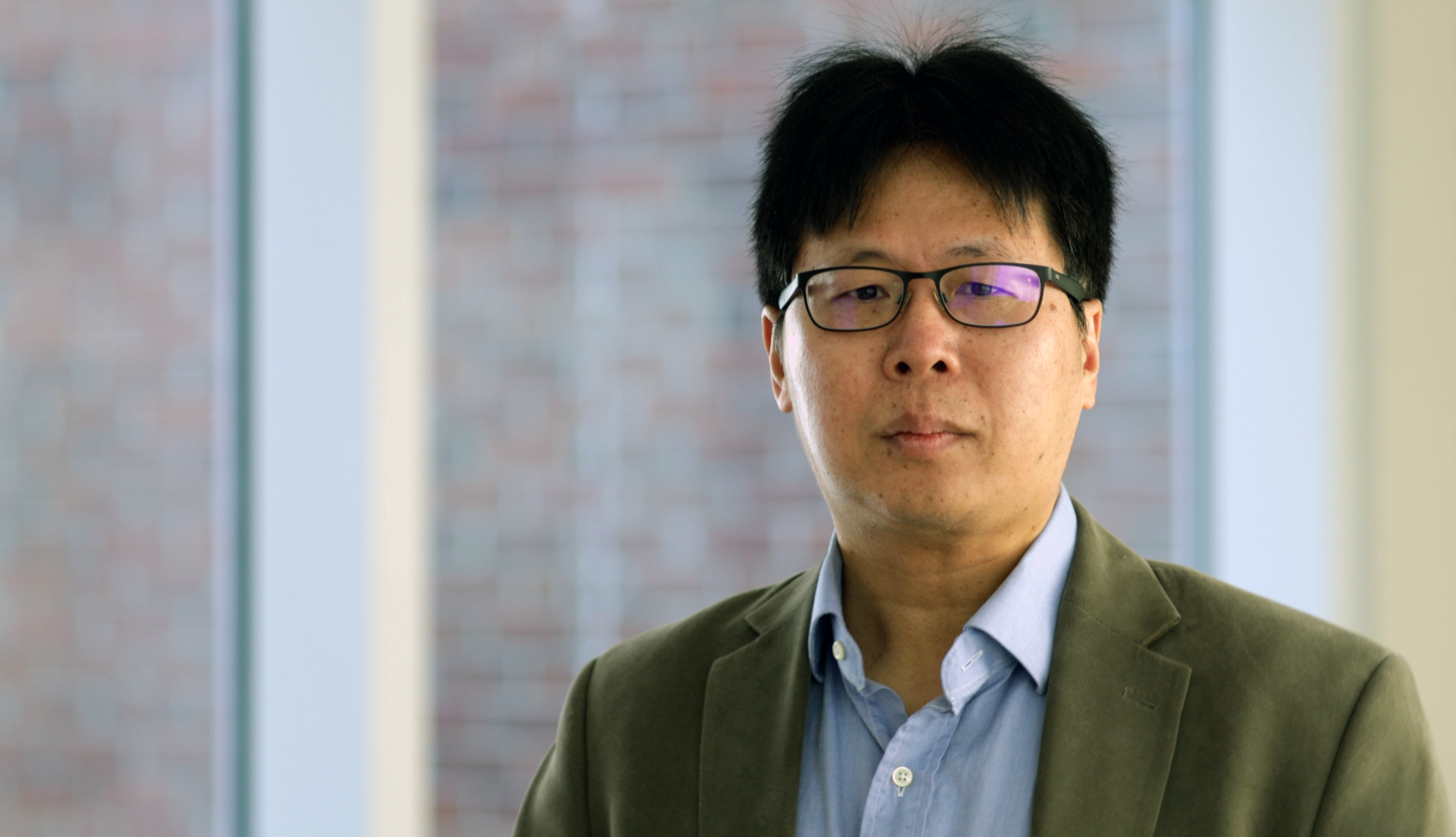
Transforming cancer diagnosis: AI model offers greater accuracy
Researchers at the University of Maine have developed an advanced artificial intelligence (AI) model to improve breast cancer diagnosis by mimicking how pathologists analyze tissue samples.
The new Context Guided Segmentation Network (CGS-Net) provides more accurate cancer detection, addressing key limitations in current diagnostic methods. The study that resulted in its creation, “Context-Guided Segmentation for Histopathologic Cancer Segmentation,” was published in Scientific Reports with Springer Nature. It is the result of a collaborative effort led by Yifeng Zhu, chair and Norman Stetson Professor of Electrical and Computer Engineering, and the team at his Data Engineering and AI Lab (DEAL).
Zhu began applying modern AI technology for cancer detection in 2018. While caring for his mother during chemotherapy in 2017, he noticed the challenges pathologists face when reviewing biopsy samples under a microscope — an often tedious and error-prone process. Motivated by this firsthand experience, Zhu sought to streamline and improve diagnostic accuracy by leveraging advanced AI techniques, ultimately leading to the creation of CGS-Net.
His research group also participated in the international competition Liver Cancer Segmentation Challenge and ranked in the top 10 among all teams in 2019, with the results published in the journal Medical Image Analysis.
This research is an interdisciplinary collaboration with Chaofan Chen, assistant professor of computer science, and Andre Khalil, professor of chemical and biomedical engineering and Ph.D. students Jeremy Juybari and Josh Hamilton. It explores a new AI model inspired by the way pathologists navigate slides under a microscope, zooming in and out to gather both broad context and detailed information. Because pathologists rely on contextual cues to identify and evaluate abnormalities, this model mimics that approach — incorporating surrounding context while also focusing on specific regions of interest.
Breast cancer is the second leading cause of cancer-related deaths among women, and its diagnosis depends heavily on the microscopic examination of stained tissue samples. However, limited access to trained pathologists, particularly in under-resourced regions, contributes to diagnostic delays. CGS-Net has the potential to assist pathologists, especially in areas with fewer healthcare resources, by identifying cancerous regions more efficiently.
“This research could significantly reduce diagnostic delays, especially in under-resourced regions where access to trained pathologists is limited,” said Jeremy Juybari, the first author of this paper and Ph.D. student in electrical and computer engineering.
CGS-Net is a dual-encoder deep learning model that simultaneously evaluates tissue at different magnification levels. Unlike traditional models, which analyze tissue at a single resolution, CGS-Net incorporates both detailed and contextual views, mimicking how pathologists zoom in and out during their examinations. This leads to more precise cancer segmentation and improved diagnostic accuracy.
Tested on the Camelyon16 dataset, which includes sentinel lymph node tissue samples, CGS-Net showed significant improvements in precise breast cancer detection over traditional models, with an area under the curve increase of 0.92% and a cancer Dice score improvement of 6.81%. These results highlight the model’s effectiveness in reducing false positives and improving accuracy.
The technical foundation of CGS-Net is a transformer dual-encoder architecture that incorporates cross-attention mechanisms to integrate detailed and contextual views. Unlike existing multi-resolution models, CGS-Net uniquely initializes cross-attention weights to enhance information sharing between magnification levels. The system was trained in two phases: first by optimizing the detail and context encoders separately, then by integrating them into a joint training model.
Additionally, the research introduced a robust patch-extraction algorithm to standardize data inputs, ensuring consistency and reproducibility in machine learning models for whole-slide imaging datasets. CGS-Net was rigorously evaluated using MiT and Swin V2 encoders, further validating its performance across various architectures and datasets.
“Our research goal is not to replace pathologists,” said Zhu. “Instead, we want to complement their expertise by providing an AI tool to assist them.”